在簡單線性迴歸中,根據高斯-馬可夫定理 (Gauss-Markov Theorem)
只要殘差符合以下的假設,我們使用一般最小平方法來估計Beta時,
就可以得到具有~最佳線性不偏估計量(BLUE)的性質.
迴歸模型的殘差 (residual) 必需符合以下的性質 (古典迴歸的假設)
1. 殘差期望值為零 (zero mean) : E(u) = 0
2. 殘差具同質變異 (homoskedasticity) : var(u) = σ^2,σ^2為constant
3. 殘差無自我相關 (non-autocorrelation) : cov(ut, ut-s) = 0, for s ≠0
4. 自變數與殘差無相關 (orthogonality) : cov(x, u) = 0, for any i
* 文獻上亦將符合以上要求之殘差稱為獨立相同分配(iid)殘差
5. 殘差為常態性 (normality)
* 如果簡單線性迴歸殘差亦符合以上第 (5) 個假設,則 OLS 所得到之估計式也將具有常態分配的性質,不過只要樣本數夠大,OLS 所得到估計式之分配將可漸進為常態分配。所以其重要性在樣本數多的時候就相對較不重要。
使用 OLS 估計時BLUE 性質的重要性:
殘差若不符合高斯-馬可夫定理的要求,則我們用 OLS 所估得之係數,就不一定會具有 BLUE 的性質。根據沒有BLUE 性質的迴歸係數進行統計決策或推論,在實務應用上會有較高的錯誤機率。
所以正確的步驟應該為:
使用 OLS 進行估計時,應該先檢查殘差是否符合高斯-馬可夫定理對迴歸殘差的假設要求,通過符合假設之檢定後,再對迴歸係數進行統計推論才是正確的迴歸分析步驟。
*殘差具同質變異 (homoskedasticity)及自變數與殘差無相關(orthogonality) (假設(2)及假設(4))
a. 變異數不同質之原因,來自殘差與自變數相關,又可稱為比例異質性 (proportional heteroskedasticity) –常用White’s test
b. 變異數不同質之原因,來自變異數和殘差落後期平方相關,又稱為自我相關異質變異 (Autoregressive Conditional Heterorscedasticity, ARCH) –常用ARCH-LM 檢定及Q 平方檢定
*殘差無自我相關 (non-autocorrelation) (假設(3))
殘差間是否存在自我相關的性質 –常用Q 檢定進行檢定
*殘差之期望值是否為零 (zero mean) (假設(1))
由於使用OLS 估計之殘差大多很接近
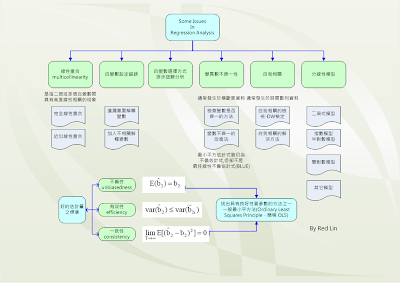